Author: a Shui, member of Datawhale, Beijing University of Aeronautics and Astronautics
Based on the handwritten OCR recognition competition of the world AI innovation competition (AIWIN), this paper gives the common ideas and processes of OCR practice. This project uses the PaddlePaddle 2 dynamic diagram to implement the CRNN character recognition model.
Code address: https://aistudio.baidu.com/aistudio/projectdetail/2612313

Competition background
The bank's daily business involves the identification and entry of various vouchers, such as ID card entry, check entry, statement entry, etc. In the past, the input method was mainly manual, which was inefficient and labor cost was high. In recent years, OCR related technology is gradually replacing the traditional manual input method because of its automatic execution and less human intervention. However, there are also some problems in the practical application of OCR technology. In the recognition of various voucher fields, due to the large font difference, the number of words is not fixed, the semantic relevance is low, the voucher background interference and other reasons, the OCR recognition rate and accuracy are not high, which requires a lot of manual correction, which has a certain impact on the daily bank entry business.
Competition address: http://ailab.aiwin.org.cn/competitions/65
Competition task
The competition will provide handwritten image slice data set. The data set is obtained from the real business scenario through slice desensitization. The participating teams obtain the corresponding recognition results through recognition technology. Namely:
- Input: handwritten image slice dataset
- Output: corresponding recognition result
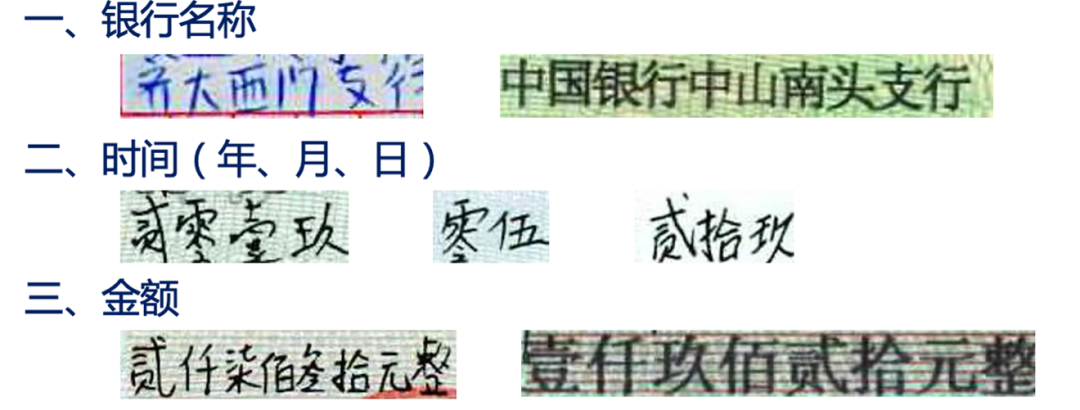
Code description
This project is a CRNN character recognition model implemented by PaddlePaddle 2 dynamic diagram, which can support different picture input. Crnn is an end-to-end recognition mode, which can complete all text recognition in the picture without dividing the picture. The structure of crnn is mainly CNN+RNN+CTC, and their respective functions are:
- Using depth CNN, the features of the input image are extracted to obtain the feature map;
- Bidirectional RNN (BLSTM) is used to predict the feature sequence, learn each feature vector in the sequence, and output the prediction label (real value) distribution;
- Using CTC Loss, a series of label distributions obtained from the loop layer are converted into the final label sequence.

The structure of CRNN is as follows: a picture with a height of 32 and an arbitrary width. After multi-layer convolution, the height of a picture becomes 1, and the height is removed after padding. Square(), that is, after convolution from the input picture BCHW, it becomes BCW. Then change the feature order from BCW to WBC and input it into RNN. After twice RNN, the final input of the model is (W, B, Class_num). This happens to be the input to the CTCLoss function.

Code details
Operating environment:
- PaddlePaddle 2.0.1
- Python 3.7
!\rm -rf __MACOSX/ Test set/ Training set/ dataset/ !unzip 2021A_T1_Task1_The data set includes training set and test set.zip > out.log
Step 1: generate additional data sets
This step can be skipped. If you want to obtain better accuracy, you can add it yourself.
import os import time from random import choice, randint, randrange from PIL import Image, ImageDraw, ImageFont # Character set of verification code picture text characters = 'RMB one hundred and five million one hundred and eighty-two yuan and sixty-nine cents and four hundred and seventy-one million yuan' def selectedCharacters(length): result = ''.join(choice(characters) for _ in range(length)) return result def getColor(): r = randint(0, 100) g = randint(0, 100) b = randint(0, 100) return (r, g, b) def main(size=(200, 100), characterNumber=6, bgcolor=(255, 255, 255)): # Create blank images and drawing objects imageTemp = Image.new('RGB', size, bgcolor) draw01 = ImageDraw.Draw(imageTemp) # Generates and calculates the width and height of a random string text = selectedCharacters(characterNumber) print(text) font = ImageFont.truetype(font_path, 40) width, height = draw01.textsize(text, font) if width + 2 * characterNumber > size[0] or height > size[1]: print('Illegal size') return # Draws characters in a random string startX = 0 widthEachCharater = width // characterNumber for i in range(characterNumber): startX += widthEachCharater + 1 position = (startX, (size[1] - height) // 2) draw01.text(xy=position, text=text[i], font=font, fill=getColor()) # Fine tune the pixel position to achieve the effect of distortion imageFinal = Image.new('RGB', size, bgcolor) pixelsFinal = imageFinal.load() pixelsTemp = imageTemp.load() for y in range(size[1]): offset = randint(-1, 0) for x in range(size[0]): newx = x + offset if newx >= size[0]: newx = size[0] - 1 elif newx < 0: newx = 0 pixelsFinal[newx, y] = pixelsTemp[x, y] # Draw interference pixels with random colors and random positions draw02 = ImageDraw.Draw(imageFinal) for i in range(int(size[0] * size[1] * 0.07)): draw02.point((randrange(0, size[0]), randrange(0, size[1])), fill=getColor()) # Save and display pictures imageFinal.save("dataset/images/%d_%s.jpg" % (round(time.time() * 1000), text)) def create_list(): images = os.listdir('dataset/images') f_train = open('dataset/train_list.txt', 'w', encoding='utf-8') f_test = open('dataset/test_list.txt', 'w', encoding='utf-8') for i, image in enumerate(images): image_path = os.path.join('dataset/images', image).replace('\\', '/') label = image.split('.')[0].split('_')[1] if i % 100 == 0: f_test.write('%s\t%s\n' % (image_path, label)) else: f_train.write('%s\t%s\n' % (image_path, label)) def creat_vocabulary(): # Generate vocabulary with open('dataset/train_list.txt', 'r', encoding='utf-8') as f: lines = f.readlines() v = set() for line in lines: _, label = line.replace('\n', '').split('\t') for c in label: v.add(c) vocabulary_path = 'dataset/vocabulary.txt' with open(vocabulary_path, 'w', encoding='utf-8') as f: f.write(' \n') for c in v: f.write(c + '\n') if __name__ == '__main__': if not os.path.exists('dataset/images'): os.makedirs('dataset/images')
Step 2: install the dependent environment
!pip install Levenshtein Looking in indexes: https://pypi.tuna.tsinghua.edu.cn/simple Requirement already satisfied: Levenshtein in /opt/conda/envs/python35-paddle120-env/lib/python3.7/site-packages (0.16.0) Requirement already satisfied: rapidfuzz<1.9,>=1.8.2 in /opt/conda/envs/python35-paddle120-env/lib/python3.7/site-packages (from Levenshtein) (1.8.2)
Step 3: read the dataset
import glob, codecs, json, os import numpy as np date_jpgs = glob.glob('./Training set/date/images/*.jpg') amount_jpgs = glob.glob('./Training set/amount/images/*.jpg') lines = codecs.open('./Training set/date/gt.json', encoding='utf-8').readlines() lines = ''.join(lines) date_gt = json.loads(lines.replace(',\n}', '}')) lines = codecs.open('./Training set/amount/gt.json', encoding='utf-8').readlines() lines = ''.join(lines) amount_gt = json.loads(lines.replace(',\n}', '}'))
data_path = date_jpgs + amount_jpgs date_gt.update(amount_gt) s = '' for x in date_gt: s += date_gt[x] char_list = list(set(list(s))) char_list = char_list
Step 4: construct training set
!mkdir dataset !mkdir dataset/images !cp Training set/date/images/*.jpg dataset/images !cp Training set/amount/images/*.jpg dataset/images mkdir: cannot create directory 'dataset': File exists mkdir: cannot create directory 'dataset/images': File exists
with open('dataset/vocabulary.txt', 'w') as up: for x in char_list: up.write(x + '\n') data_path = glob.glob('dataset/images/*.jpg') np.random.shuffle(data_path) with open('dataset/train_list.txt', 'w') as up: for x in data_path[:-100]: up.write(f'{x}\t{date_gt[os.path.basename(x)]}\n') with open('dataset/test_list.txt', 'w') as up: for x in data_path[-100:]: up.write(f'{x}\t{date_gt[os.path.basename(x)]}\n')
The pictures generated by executing the above program will be placed in the dataset/images directory, and the generated training data list and test data list will be placed in dataset / train respectively_ List.txt and dataset/test_list.txt, and finally a data vocabulary dataset/vocabulary.txt.
The format of the data list is as follows: the path of the picture is on the left and the text label is on the right.
dataset/images/1617420021182_c1dw.jpg c1dw dataset/images/1617420021204_uvht.jpg uvht dataset/images/1617420021227_hb30.jpg hb30 dataset/images/1617420021266_4nkx.jpg 4nkx dataset/images/1617420021296_80nv.jpg 80nv
The following is the format of the dataset vocabulary, one character per line, and the first line is a space, which does not represent any characters.
f s 2 7 3 n d w
For training user-defined data, refer to the above format.
Step 5: Training Model
Whether you customize the dataset or use the data generated above, as long as the file path is correct, you can start training. The training supports image input with different lengths, but the data length of each batch should be the same. In this case, the author uses collate_fn() function, which can find the longest data, and then fill other data with 0 and add it to the same length. At the same time, the function also outputs the actual length of each data label in it, because the loss function needs to input the actual length of the label.
- During the training process, the program will use VisualDL to record the training results
import paddle import numpy as np import os from datetime import datetime from utils.model import Model from utils.decoder import ctc_greedy_decoder, label_to_string, cer from paddle.io import DataLoader from utils.data import collate_fn from utils.data import CustomDataset from visualdl import LogWriter # Training data list path train_data_list_path = 'dataset/train_list.txt' # Test data list path test_data_list_path = 'dataset/test_list.txt' # Glossary path voc_path = 'dataset/vocabulary.txt' # Path to model save save_model = 'models/' # Data size of each batch batch_size = 32 # Pre training model path pretrained_model = None # Number of training rounds num_epoch = 100 # Initial learning rate learning_rate = 1e-3 # Logging writer = LogWriter(logdir='log') def train(): # Get training data train_dataset = CustomDataset(train_data_list_path, voc_path, img_height=32) train_loader = DataLoader(dataset=train_dataset, batch_size=batch_size, collate_fn=collate_fn, shuffle=True) # Get test data test_dataset = CustomDataset(test_data_list_path, voc_path, img_height=32, is_data_enhance=False) test_loader = DataLoader(dataset=test_dataset, batch_size=batch_size, collate_fn=collate_fn) # Get model model = Model(train_dataset.vocabulary, image_height=train_dataset.img_height, channel=1) paddle.summary(model, input_size=(batch_size, 1, train_dataset.img_height, 500)) # Set optimization method boundaries = [30, 100, 200] lr = [0.1 ** l * learning_rate for l in range(len(boundaries) + 1)] scheduler = paddle.optimizer.lr.PiecewiseDecay(boundaries=boundaries, values=lr, verbose=False) optimizer = paddle.optimizer.Adam(parameters=model.parameters(), learning_rate=scheduler, weight_decay=paddle.regularizer.L2Decay(1e-4)) # Get loss function ctc_loss = paddle.nn.CTCLoss() # Load pre training model if pretrained_model is not None: model.set_state_dict(paddle.load(os.path.join(pretrained_model, 'model.pdparams'))) optimizer.set_state_dict(paddle.load(os.path.join(pretrained_model, 'optimizer.pdopt'))) train_step = 0 test_step = 0 # Start training for epoch in range(num_epoch): for batch_id, (inputs, labels, input_lengths, label_lengths) in enumerate(train_loader()): out = model(inputs) # Calculate loss input_lengths = paddle.full(shape=[batch_size], fill_value=out.shape[0], dtype='int64') loss = ctc_loss(out, labels, input_lengths, label_lengths) loss.backward() optimizer.step() optimizer.clear_grad() # Multi card training uses only one process to print if batch_id % 100 == 0: print('[%s] Train epoch %d, batch %d, loss: %f' % (datetime.now(), epoch, batch_id, loss)) writer.add_scalar('Train loss', loss, train_step) train_step += 1 # Execution evaluation if epoch % 10 == 0: model.eval() cer = evaluate(model, test_loader, train_dataset.vocabulary) print('[%s] Test epoch %d, cer: %f' % (datetime.now(), epoch, cer)) writer.add_scalar('Test cer', cer, test_step) test_step += 1 model.train() # Record learning rate writer.add_scalar('Learning rate', scheduler.last_lr, epoch) scheduler.step() # Save model paddle.save(model.state_dict(), os.path.join(save_model, 'model.pdparams')) paddle.save(optimizer.state_dict(), os.path.join(save_model, 'optimizer.pdopt')) # Evaluation model def evaluate(model, test_loader, vocabulary): cer_result = [] for batch_id, (inputs, labels, _, _) in enumerate(test_loader()): # Execution identification outs = model(inputs) outs = paddle.transpose(outs, perm=[1, 0, 2]) outs = paddle.nn.functional.softmax(outs) # Decoding to obtain recognition results labelss = [] out_strings = [] for out in outs: out_string = ctc_greedy_decoder(out, vocabulary) out_strings.append(out_string) for i, label in enumerate(labels): label_str = label_to_string(label, vocabulary) labelss.append(label_str) for out_string, label in zip(*(out_strings, labelss)): # Calculate word error rate c = cer(out_string, label) / float(len(label)) cer_result.append(c) cer_result = float(np.mean(cer_result)) return cer_result if __name__ == '__main__': train()
Step 6: model prediction
After the training, the saved model is used for prediction. By modifying the image_path specifies the picture path to be predicted and the decoding method. The author uses the simplest greedy strategy.
import os from PIL import Image import numpy as np import paddle from utils.model import Model from utils.data import process from utils.decoder import ctc_greedy_decoder with open('dataset/vocabulary.txt', 'r', encoding='utf-8') as f: vocabulary = f.readlines() vocabulary = [v.replace('\n', '') for v in vocabulary] save_model = 'models/' model = Model(vocabulary, image_height=32) model.set_state_dict(paddle.load(os.path.join(save_model, 'model.pdparams'))) model.eval() def infer(path): data = process(path, img_height=32) data = data[np.newaxis, :] data = paddle.to_tensor(data, dtype='float32') # Execution identification out = model(data) out = paddle.transpose(out, perm=[1, 0, 2]) out = paddle.nn.functional.softmax(out)[0] # Decoding to obtain recognition results out_string = ctc_greedy_decoder(out, vocabulary) # print('forecast result:% s'% out_string) return out_string if __name__ == '__main__': image_path = 'dataset/images/0_8bb194207a248698017a854d62c96104.jpg' display(Image.open(image_path)) print(infer(image_path))
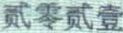
<PIL.JpegImagePlugin.JpegImageFile image mode=RGB size=123x33 at 0x7F4D525F08D0> Two zero two one
from tqdm import tqdm, tqdm_notebook result_dict = {} for path in tqdm(glob.glob('./Test set/date/images/*.jpg')): text = infer(path) result_dict[os.path.basename(path)] = { 'result': text, 'confidence': 0.9 } for path in tqdm(glob.glob('./Test set/amount/images/*.jpg')): text = infer(path) result_dict[os.path.basename(path)] = { 'result': text, 'confidence': 0.9 }
with open('answer.json', 'w', encoding='utf-8') as up: json.dump(result_dict, up, ensure_ascii=False, indent=4) !zip answer.json.zip answer.json adding: answer.json (deflated 85%)